The Evolving Landscape of AI: Beyond Algorithms and Hardware

Artificial Intelligence (AI) has long been synonymous with cutting-edge algorithms and high-performance hardware. Companies and researchers have poured immense resources into developing these world-class AI technologies to ensure optimized performance and innovation. However, a new paradigm is emerging – the focus is shifting towards gathering unbiased, holistic, and comprehensive data from multiple sources. This shift is crucial as the data utilized by AI becomes the backbone for accurate and reliable decision-making.
The Importance of Quality Data in AI
AI algorithms are only as good as the data fed into them. Without high-quality, unbiased, and comprehensive data, even the most sophisticated AI can produce flawed or biased outputs. This realization has led the industry to pay closer attention to data acquisition and management.
One striking example of the importance of quality data in AI is in medical diagnostics. AI systems, trained on diverse and comprehensive datasets from various demographics and medical histories, can detect diseases like cancer with higher accuracy. For instance, an AI developed for breast cancer detection demonstrated an impressive success rate when trained on a diversified dataset comprising mammograms from different age groups and ethnic backgrounds. In contrast, another AI trained on a limited and homogeneous dataset, missed critical diagnoses for minority groups. This stark difference underscores that the efficacy and fairness of AI in sensitive applications such as healthcare are heavily dependent on the inclusiveness and accuracy of the data involved.
Elon Musk, renowned for his work in AI and technology, once said, "AI is probably the most important thing humanity has ever worked on. But misaligned AI could be far worse than nukes." This ties directly into the importance of data quality – if the foundational data is skewed or limited, the repercussions could be significant.
Unbiased Data: Ensuring Fairness and Accuracy
An unbiased dataset ensures that AI systems do not perpetuate existing stereotypes or biases. This is especially critical in applications like hiring algorithms, loan approval systems, and even criminal justice. By sourcing data from diverse and multiple sources, AI systems can better represent and serve all segments of the population fairly.
"A well-rounded and unbiased dataset is paramount for creating fair AI systems. We must be diligent in our data collection practices to pave the way for ethical AI development," says Sundar Pichai, CEO of Alphabet Inc.
A compelling real-world example that underscores the importance of unbiased data in ensuring fairness and accuracy can be witnessed in the realm of facial recognition technology. Several high-profile studies have revealed that many facial recognition systems exhibit a significant bias against people of color, particularly Black women, who are misidentified at alarmingly higher rates than their white counterparts. This discrepancy stems from training datasets that predominantly consist of images of lighter-skinned individuals, leading to an inherent bias in the algorithms.
For example, the National Institute of Standards and Technology (NIST) conducted a study that found that many commercial facial recognition systems produce false positives for Asian and African American faces at rates of 10 to 100 times higher than for Caucasian faces. This reality calls for a deliberate effort to include a diverse array of demographic information in the training datasets to mitigate bias and improve the accuracy of these AI systems. As a result, tech companies are now striving to create more inclusive and representative datasets, ultimately aiming to develop fairer and more reliable facial recognition technologies.
Holistic Data: Capturing the Full Picture
Holistic data encompasses a wide range of variables and scenarios, offering a comprehensive view of the context in which AI operates. This is essential for creating AI systems that can adapt to real-world complexities and nuances.
An example of this would be holistic data's role in ethical decision-making in the financial sector, particularly in the context of bank loans. AI models utilizing comprehensive datasets that include a wide array of variables—such as applicants' full financial histories, socio-economic backgrounds, employment trajectories, and even local economic conditions—can make more equitable and accurate lending decisions. Analyzing holistic data ensures that AI systems do not unjustly penalize individuals who may not fit traditional creditworthiness metrics but have strong potential for loan repayment. This prevents systemic biases and promotes financial inclusion by offering fair loan opportunities to a broader population. Larry Fink, CEO of BlackRock, aptly stated, "Inclusive datasets are essential for AI to foster financial equity and growth, ensuring we build an economy that serves everyone." By capturing the complete picture, holistic data helps AI models in the banking sector deliver decisions that are not only economically sound but also ethically responsible.
Demis Hassabis, co-founder of DeepMind, highlights this by stating, "The essence of AI's potential lies in its ability to adapt and learn from full-spectrum data. The more holistic our approach, the more capable and relevant our AI models become."
Comprehensive Data: Bridging the Gaps
To develop AI systems that are both effective and reliable, comprehensive data collection is critical. Ensuring data comprehensiveness means gathering information from a variety of sources and formats, spanning different timespans and conditions. This not only enhances the predictive power of AI models but also minimizes the risk of overlooking crucial information.
Temporal diversity in datasets ensures that AI systems can recognize patterns and anomalies over long periods and adjust to temporal shifts, such as seasonal trends and evolving market conditions. Similarly, data sourced from various environmental and situational contexts enables AI to operate effectively under a range of circumstances, from normal to extreme scenarios.
For instance, in weather prediction, training AI models on meteorological data from different seasons, climatic events, and geographic locations allows for accurate forecasting irrespective of the conditions. This comprehensive approach helps prevent models from being overly sensitive to short-term fluctuations or specific situations, thereby improving their generalizability and stability. That said, integrating a wide array of timespans and conditions into training datasets is crucial for building AI systems that are not only accurate but also adaptive and resilient.
"Inaccurate or incomplete data will lead to poor AI decisions. A comprehensive data strategy is not just a best practice but a necessity," asserts Fei-Fei Li, a leading AI researcher and professor at Stanford University.
Reliable Data
The landscape of AI is rapidly evolving, with a significant shift in focus towards the quality and comprehensiveness of the data driving these intelligent systems. While world-class algorithms and cutting-edge hardware remain important, the future of AI hinges on our ability to gather and utilize unbiased, holistic, and comprehensive datasets. This approach ensures fair, accurate, and impactful AI outcomes, ultimately paving the way for a more ethical and effective AI-enhanced world.
As industry giants and thought leaders in AI affirm, "Data is the lifeblood of AI." Ensuring its quality and comprehensiveness is not just a step forward but an essential leap towards realizing the full potential of artificial intelligence.
Recommended to you




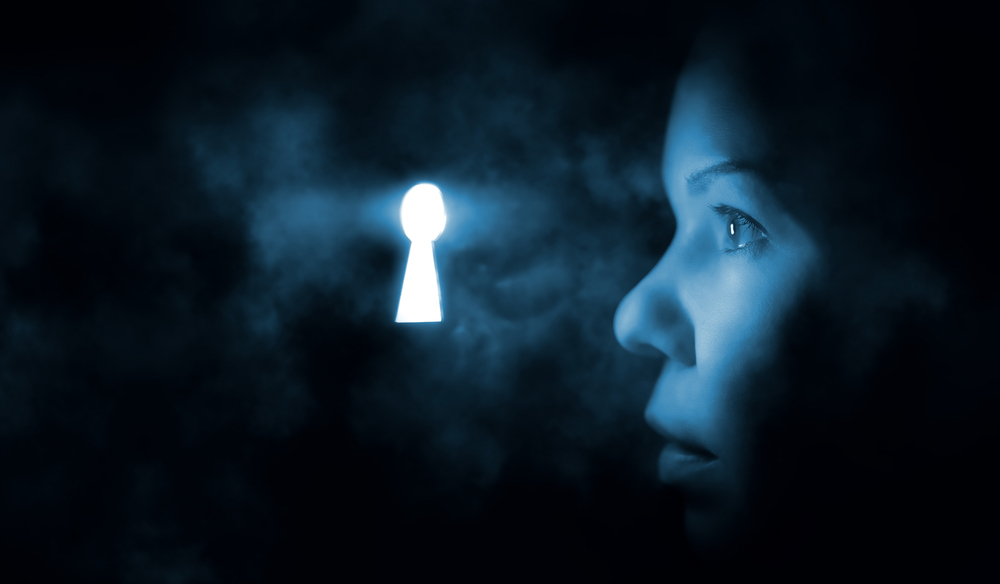
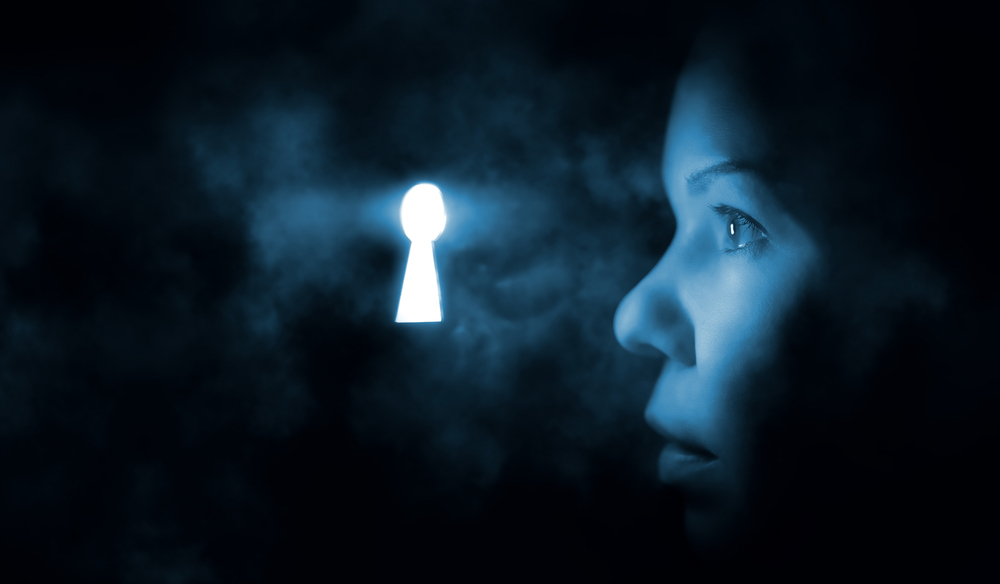